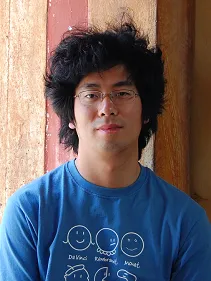
Meng Lu
Peking University
# Time
Tues., 14:30-15:30
Oct. 15, 2024
# Venue
Jingzhai 105
#Abstract
Understanding intelligence has long been a central pursuit in neuroscience, cognitive science, and artificial intelligence. It encompasses complex phenomena such as learning, problem-solving, creativity, and consciousness. While recent advancements in geometric analysis have shed light on the representation and organization of high-dimensional information in neural and artificial systems, a comprehensive framework that unifies the static and dynamic aspects of intelligence remains elusive. In this talk, I will introduce a novel mathematical framework based on Riemannian geometry that models both the structure and dynamics of intelligence and consciousness.
In this framework, elements of intelligence are conceptualized as tokens embedded in a high-dimensional space. These token embeddings capture the relationships between various scenarios and tasks, forming manifolds in the intelligence space. Thought flow is represented as the sequential activation of tokens along geodesics within these manifolds. Consciousness, as a self-referential process, perceives and evaluates the thought flow, providing feedback through prediction errors. These errors adjust the geodesic paths, restructuring the manifolds and facilitating learning. This dynamic interaction between intelligence and consciousness guides the evolution of their underlying geometry, offering new insights into both biological and artificial intelligence systems. This unified framework opens avenues for future research and empirical validation. Altogether, this framework can be summarised as “geometry dictates how consciousness navigates, while consciousness guides the evolution of geometry.”