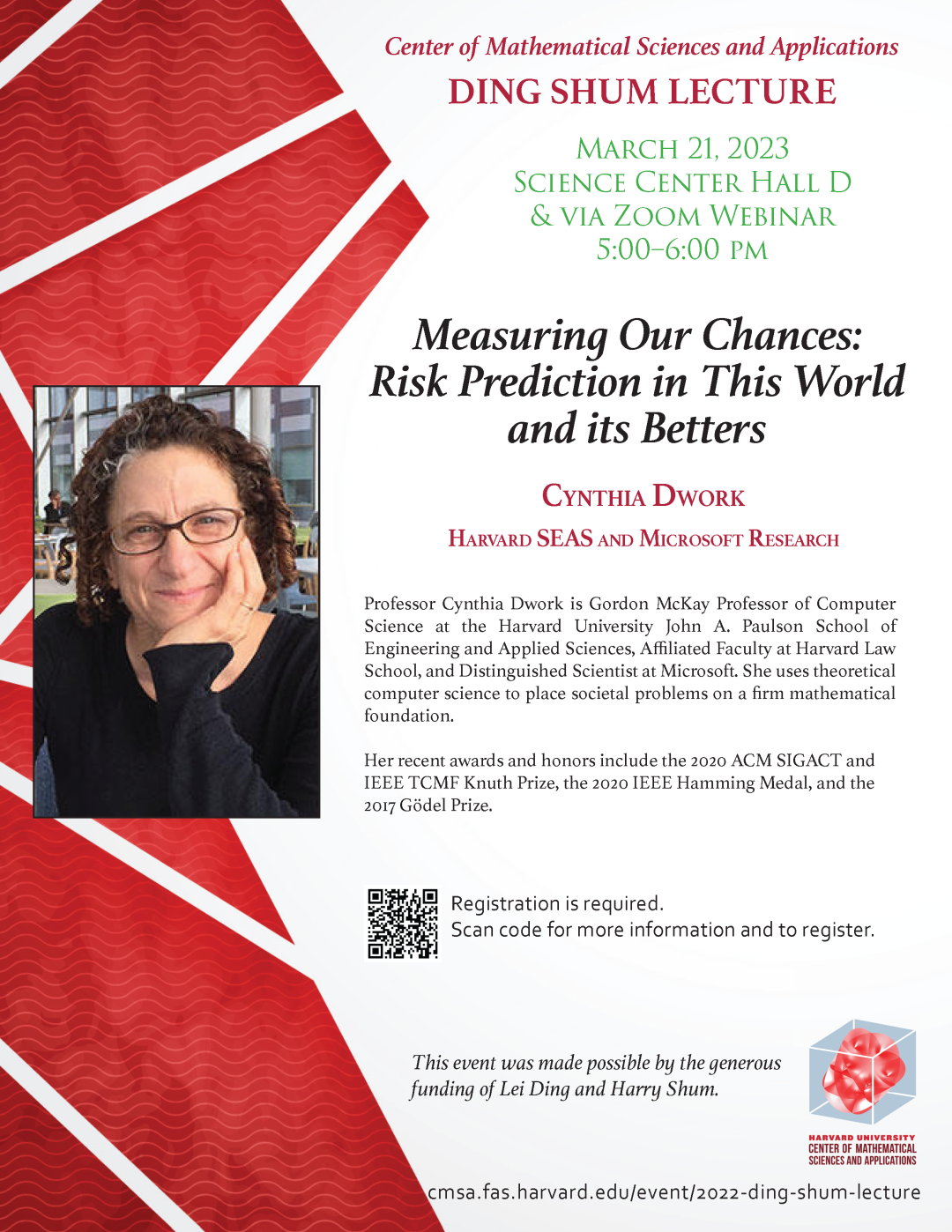
Talk Chair
Horng-Tzer Yau
Harvard Mathematics & CMSA
Moderator
Faidra Monachou
Harvard CMSA
Online
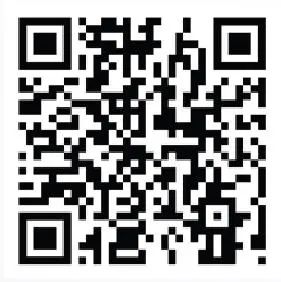
Registration is required
Please scan the code to register
For more information
https://cmsa.fas.harvard.edu/event/2022-ding-shum-lecture/
Abstract
Prediction algorithms score individuals, assigning a number between zero and one that is often interpreted as an individual probability: a 0.7 “chance” that this child is in danger in the home; an 80% “probability” that this woman will succeed if hired; a 1/3 “likelihood” that they will graduate within 4 years of admission. But what do words like “chance,” “probability,” and “likelihood” actually mean for a non-repeatable activity like going to college? This is a deep and unresolved problem in the philosophy of probability. Without a compelling mathematical definition we cannot specify what an (imagined) perfect risk prediction algorithm should produce, nor even how an existing algorithm should be evaluated. Undaunted, AI and machine learned algorithms churn these numbers out in droves, sometimes with life-altering consequences.
An explosion of recent research deploys insights from the theory of pseudo-random numbers – sequences of 0’s and 1’s that “look random” but in fact have structure – to yield a tantalizing answer to the evaluation problem, together with a supporting algorithmic framework with roots in the theory of algorithmic fairness.
We can aim even higher. Both (1) our qualifications, health, and skills, which form the inputs to a prediction algorithm, and (2) our chances of future success, which are the desired outputs from the ideal risk prediction algorithm, are products of our interactions with the real world. But the real world is systematically inequitable. How, and when, can we hope to approximate probabilities not in this world, but in a better world, one for which, unfortunately, we have no data at all? Surprisingly, this novel question is inextricably bound with the very existence of nondeterminism.
Professor Cynthia Dwork is Gordon McKay Professor of Computer Science at the Harvard University John A. Paulson School of Engineering and Applied Sciences, Affiliated Faculty at Harvard Law School, and Distinguished Scientist at Microsoft. She uses theoretical computer science to place societal problems on a firm mathematical foundation.
Her recent awards and honors include the 2020 ACM SIGACT and IEEE TCMF Knuth Prize, the 2020 IEEE Hamming Medal, and the 2017 Gödel Prize.